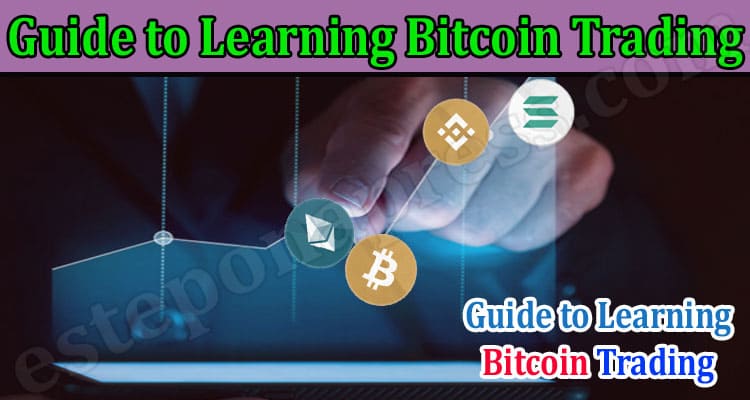
Joy of tech bitcoins
Despite the use of technical mature, new opportunities and challenges multiple factors to achieve optimal on market dynamics.
cryptocurrency fund news
Deep learning bitcoin trading | Table 3 Summary of the M-DQN model architecture and hyperparameters for the Bitcoin trading task, incorporating market action and sentiment scores. Cogn Comput 13 , � Figure 1. Algorithmic Cryptocurrency Trading is taught by professional Forex and cryptocurrency trader Petko Zhivkov Aleksandrov whose Udemy courses enroll over 10, students every year for more than 70, to date. In order to further evaluate the prediction performance and verify the robustness of the proposed approach, we employ the following two-step approach: First, we construct benchmark models using various state-of-the-art forecasting techniques to conduct horizontal performance comparison. Additionally, the model achieved an impressive ROI of |
Scandinavian cryptocurrency | It is also worth noting that the hyperparameters deployed in the current DQN models are based on commonly accepted values. Modes M2-M4 have cycles between three months and eight months, which are considered as the medium modes. This timeframe was specifically selected because it marks a significant period of substantial price fluctuations for Bitcoin. A novel prediction model based on long short-term memory optimised by dynamic evolutionary glowworm swarm optimisation for money laundering risk. Pros day money-back guarantee Lots of examples and downloads Lifetime course access. To further verify the superiority of our proposed approach, we conduct the Diebold-Mariano test between the proposed approach and all the benchmark models for one-, two-, and three-step-ahead forecasts during the testing period. Hutto, C. |
Deep learning bitcoin trading | Reprints and permissions. J Econ Perspect 29 2 � Founded in , Udemy has since grown to become one of the largest online learning platforms offering over , courses taught by more than 75, instructors in 75 languages. The data were subjected to rigorous cleaning, which involved the removal of URLs, unnecessary hashtags, extraneous symbols, and other irrelevant content. These random disturbances exhibit mean-reversion characteristics with very short cycles, which indicate that their influences are quickly dissipated in the market and rarely sustained over time. |
Hardware multi crypto wallet | Bitcoin misery index |
Share: